DataLink Releases Its List of Technology Trends Shaping Healthcare in 2024
By Saurabh Bhargava, vice president of data science
As we step into 2024, the healthcare industry is gearing up for a transformative journey fueled by artificial intelligence (AI) and machine learning (ML). As the data science leader at DataLink I’m not just witnessing but actively contributing to the unfolding narrative of this digital evolution powered by AI and ML.
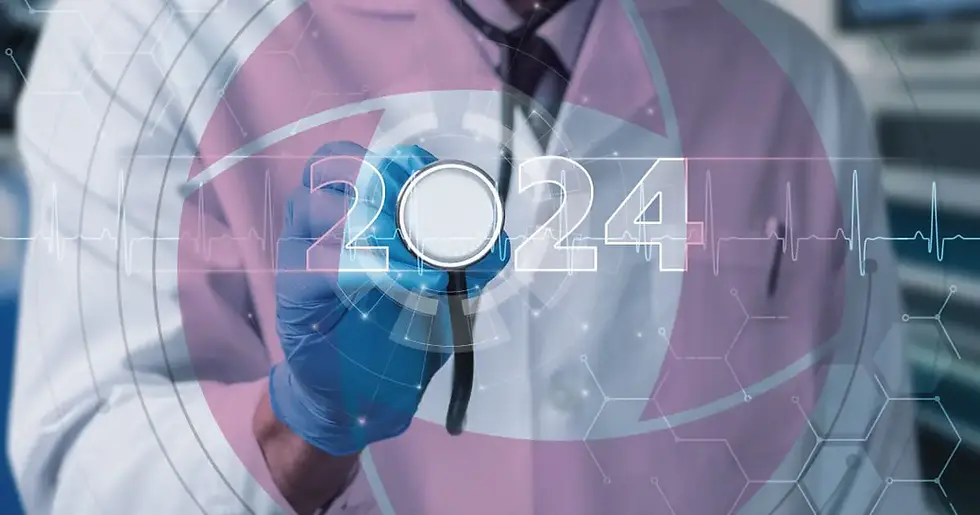
DataLink recently released its list of technology trends shaping healthcare in 2024.
“As we step into 2024, the healthcare industry is gearing up for a transformative journey fueled by AI and ML,” said Saurabh Bhargava, vice president of data science at DataLink. “In the dynamic realm of healthcare technology, the year ahead is set to maintain a continuous and ongoing focus in how we approach patient care, use the enormous amounts of data we generate, and address industry challenges.”
In “Navigating the future: Technology trends shaping healthcare in 2024,” Bhargava identified eight trends for 2024.
Predictive analytics for personalized medicine, early risk detection, and intervention strategies.
Precision healthcare with computer vision in AI-powered medical imaging.
Augmented reality and virtual reality in medical training, mental health well-being, and remote physical therapy.
Robotic process automation (RPA) streamlining administrative tasks with the power of ML.
Natural language processing (NLP) and generative AI revolutionizing clinical documentation.
IoT devices and wearables for remote patient monitoring.
Ethical AI in healthcare.
Interoperability for seamless data exchange.
“2024 promises to be an exciting year for healthcare technology. AI and ML, particularly in driving RPA and NLP solutions, aren’t just buzzwords; they’re the driving force behind a new era of innovation and efficiency in the healthcare sector,” said Bhargava. “As we embrace these trends, let's keep our focus on the goal: providing better, more accessible, and personalized healthcare for all.”
1. Predictive analytics for personalized medicine, early risk detection, and intervention strategies.
AI and ML are stepping up their game in predictive analytics, paving the way for personalized medicine. With advanced algorithms analyzing patient data, we can now tailor treatment plans based on an individual’s genetic makeup, lifestyle, and medical history.
Advances in genomics and biomarker research are enabling more personalized treatment plans and drug therapies tailored to an individual’s genetic makeup. This not only enhances patient outcomes but also optimizes resource allocation within healthcare systems.
Predictive analytics derive operational and clinical insights from real-time and historical data, and also help healthcare providers improve efficiency and act preemptively. This tailored approach allows providers to choose interventions that are more likely to be effective for a particular individual, minimizing trial-and-error in treatment selection.
2. Precision healthcare with computer vision in AI-powered medical imaging.
Computer vision empowers medical imaging solutions to interpret and analyze visual data with remarkable precision. In radiology, for instance, computer vision algorithms excel at identifying and characterizing anomalies in X-rays, CT scans, and MRIs. This accuracy translates to more reliable diagnoses and a higher level of confidence in medical imaging interpretations.
Medical imaging solutions will continue to enable early detection of abnormalities, streamline workflows for radiologists, remote diagnostics, and telemedicine along with improved surgical precision. This fusion of AI and computer vision holds the promise of a future where healthcare is more precise, accessible, and tailored to the unique needs of each patient.
3. Augmented reality and virtual reality in medical training, mental health well-being, and remote physical therapy.
The integration of augmented reality and virtual reality in medical training isn’t only transforming the way healthcare professionals learn and practice, but it’s also making significant strides in the realm of mental health and well-being. From simulated surgeries to immersive patient interaction scenarios, these technologies aren’t just bridging the gap between theory and hands-on experience; they’re also providing innovative solutions for mental health treatment.
VR therapy, for instance, is proving to be a powerful tool for addressing conditions such as anxiety, phobias, and PTSD while also offering a virtual environment for therapeutic interventions and mental health support. As we explore the potential of AR and VR, we’re not just advancing medical education but also fostering a new era of mental health care.
Similarly, these immersive technologies can bring about a paradigm shift in how physical therapy is delivered and experienced: virtual rehabilitation exercises, interactive guidance and feedback, and reducing barriers to access.
4. Robotic process automation (RPA) streamlining administrative tasks with the power of ML.
AI-driven RPA is freeing up valuable time for healthcare professionals by automating administrative tasks. Harnessing the capabilities of machine learning, RPA solutions in healthcare are becoming more adaptive and intelligent, further enhancing efficiency.
ML-driven RPA will continue to improve the healthcare administrative tasks and further enable multiple administrative tasks like prior authorization, scheduling, clinical peer-to-peer reviews, EHR documentation, or information retrieval. The synergy between RPA and ML in healthcare administration is revolutionizing routine processes and infusing intelligence through machine learning for a more agile and responsive healthcare administration that can deliver on:
Improved patient experience
Real-time analytics for next best action
Intelligent data extraction and processing
Risk mitigation, gap closure, and fraud detection
Optimizing resource allocation
5. Natural language processing (NLP) and generative AI revolutionizing clinical documentation.
The tedious task of clinical documentation is getting a makeover with the help of generative NLP. AI-driven systems, supercharged by machine learning, can now understand and extract meaningful insights from unstructured clinical notes, improving accuracy, and enabling more informed decision-making.
The recent rise of generative AI, which uses sophisticated large language models (LLMs) to create human-like text, images, and other content is driving an explosion of ideas around use cases, from reviewing medical records for accuracy to making diagnoses and treatment recommendations.
Generative AI and NLP are proving to be indispensable tools within healthcare systems, offering significant relief to providers grappling with persistent challenges. These technologies are already demonstrating their capacity to address major concerns such as escalating costs, clinician shortages, and the pervasive issue of physician burnout.
According to a study by Bain Health Systems, the highest priority use cases for generative AI in the near-term are going to be capturing, reconciliation, and structuring of patient data along with workflow optimization. Some generative AI applications are already streamlining administrative tasks and allowing thinly stretched physicians to spend more time with patients by drafting preauthorization and appeal letters.
Systems are being tested and implemented for automatically generating claims based on physician and patient discussions, auto-scheduling appointments, and referrals based on context captured from such conversations, etc. Then, there are conversational AI-based switchboards to improve the payer call center experience while reducing operators’ workload.
6. IoT devices and wearables for remote patient monitoring.
The use of mobile health apps and wearable devices for tracking fitness, health, and chronic conditions will continue to rise where AI/ML algorithms analyze the data from these devices, providing insights into trends and patterns enabling healthcare providers to make personalized recommendations for medication adjustments or lifestyle modifications, promoting proactive management of chronic conditions.
Beyond wearables, AI/ML algorithms will also improve the analysis of data from ambient technology, such as smart home devices and connected sensors which can track daily activities, sleep patterns, and environmental factors within a patient’s home and provide a comprehensive view of a patient’s well-being, supporting remote monitoring for conditions such as chronic diseases or post-operative care.
EHR-integrated AI/ML solutions can facilitate personalized patient engagement strategies and by analyzing EHR data alongside IoT-generated information. There are algorithms that can look for patterns and identify factors indicative of deteriorating health or potential complications, influencing patient behavior and adherence.
7. Ethical AI in healthcare.
Striking the right balance between innovation, privacy, and ethical practices is crucial for building trust in AI-driven healthcare solutions.
While access to PHI and adherence to HIPAA will always play in the minds of healthcare professionals, ethical considerations in AI/ML applications in healthcare are also evolving to actively address and mitigate racial and socioeconomic disparities. The emphasis on fairness, transparency, community engagement, and ongoing assessments reflects a commitment to building AI solutions that not only advance healthcare but also contribute to reducing existing disparities.
AI/ML applications in healthcare will need to evolve to consider potential biases, which can disproportionately affect certain racial or socioeconomic groups. Initiatives are being taken to ensure that datasets used to train AI models reflect the diversity of the patient population.
8. Interoperability for seamless data exchange
Breaking down data silos is a priority in 2024, ensuring that healthcare systems can seamlessly exchange information. AI and ML are being employed to facilitate interoperability, which has been a perennial challenge in healthcare, stemming from the complex and fragmented nature of most healthcare information technology and data infrastructures.
AI and ML are instrumental in achieving healthcare interoperability by standardizing data formats, ensuring semantic understanding, and facilitating seamless exchange of information. These technologies contribute to breaking down data silos, fostering collaboration among healthcare systems, and ultimately improving care coordination and patient outcomes. As interoperability continues to be a priority, the role of AI and ML in harmonizing healthcare data will become increasingly significant, which not only improves care coordination but also contributes to a more holistic view of patient health.
Comments